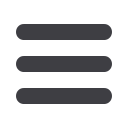
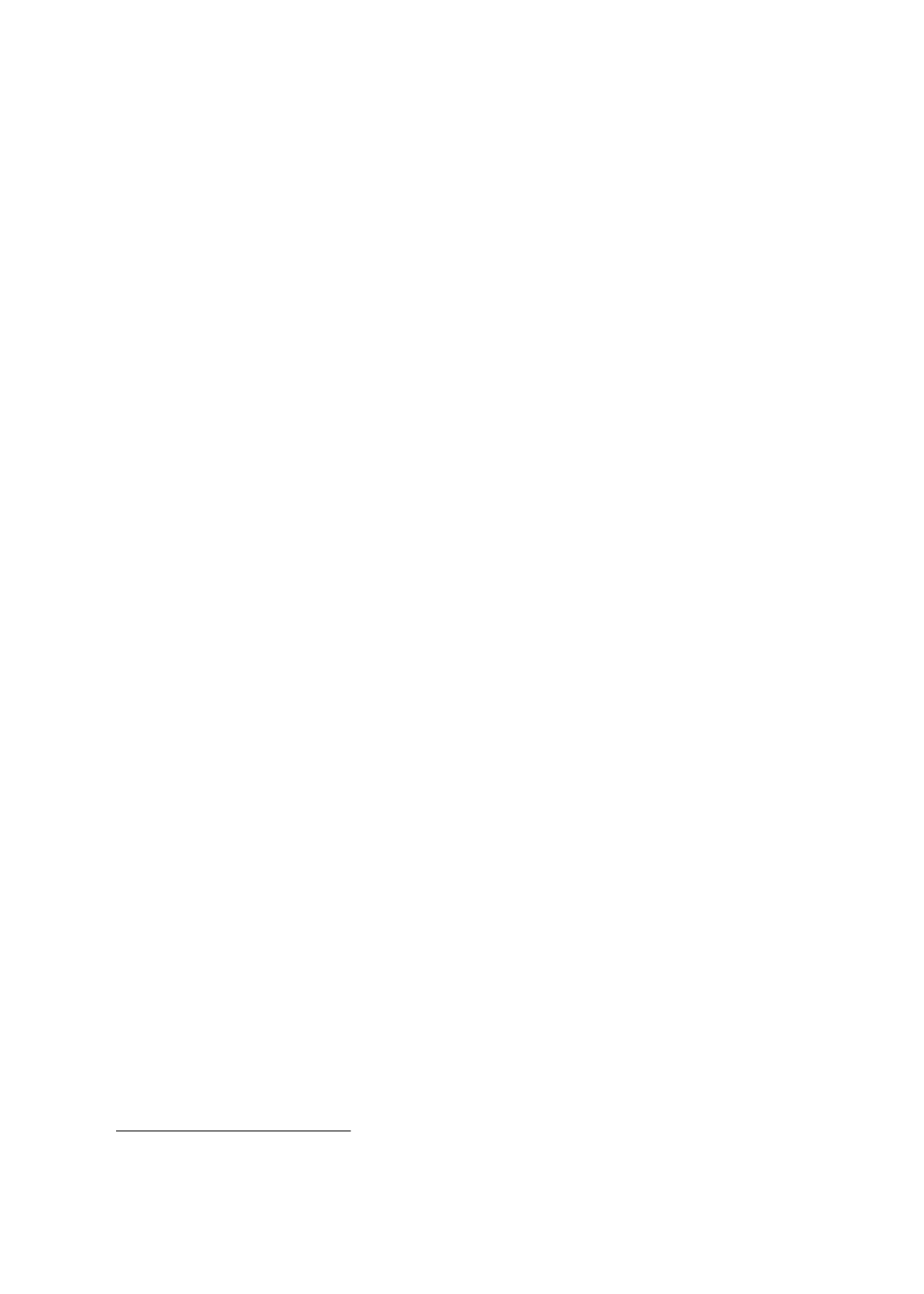
109
attempted to answer this question. It is found that in PISA 2012, differences in students’ socio-
economic backgrounds including family wealth do not account for the performance difference
between Malaysian and Singaporean students. The same conclusion also holds when compared
to South Korean students. In contrast, children from Vietnam perform at the same level as those
from Korea after accounting for socio-economic differences (Asadullah, Pereira and Xiao 2017).
This implies that economic development, poverty reduction, and income growth alone will not
close the learning shortfalls between Malaysia and other high performing East Asian countries.
3.2.6.
Regression Analysis of the Determinants of Learning Outcomes
Educational production function analyses the relationship between inputs and outputs. In this
context, education production function is usually used to determine the relationship between
school level factors that determine students’ performance. School level inputs can be class size,
teacher quality, school resources such as library, computer, teaching and learningmaterials. The
commonly used output is students’ achievement (Pigott, Williams, Pollanin & Wu-Bohanan,
2012). According to Mat Saad, Nik Yusoff & Mohammad Yassin (2001), the classroom
environment plays an important role in providing a convenient and conducive learning
environment. Small classrooms with overcrowded students and inadequate facilities make it
difficult for the learning process (Tanner & Lackney, 2006).
Conducive learning environment, smaller class size, quality and effective teachers have been
commonly cited in policy documents and literature as determinants of students’ performance.
However, recent emphasis has been on improvement on teaching quality, teaching and learning
on higher order thinking skills, promoting school culture as learning organization, school
leadership, parental commitment and encourage private sector involvement. In this section, we
discuss trends in some of these indicators based on available data and education statistics.
Table 3.2.4
presents ordinary least squares (OLS) estimates of the student achievement
function for Malaysia in Reading, Math and Science in PISA 2012 data where achievement is
examined in relation to individual, family and school factors.
28
The estimationmethods accounts
for multiple plausible values since PISA data does not report a single test score for study
subjects. Among household-specific factors, a number of results are noteworthy. Student
achievement is most sensitive to family wealth in case of mathematics scores – compared to
children from bottom 25% of the wealth distribution, children in the top 25% wealth group
enjoy an extra 44 points in PISA math score, which equivalent to nearly one extra year of
schooling. Equally, children of educated parents perform significantly better compared to those
whose parents have only lower secondary education or below. Second, while there is no
advantage to attending a private school, experience of pre-school attendance is significantly
associated with higher performance in all PISA subjects.
Among individual level factors, one notable finding is the female advantage in science and
language and the absence of any gender gap in mathematics in Malaysia. In other words,
compared to many other parts of the world where girls lag behind boys in educational
achievement, they excel in all domains of learning in Malaysia. However, the girl-boy gap in
Reading is very high and is a concern. If test language is spoken at home, this positively
influences mathematics and science scores though the correlation is negative in case of reading
score. This is important considering the fact that a large proportion of Malaysian students don’t
28
In the PISA 2012 round, 5197 students from 164 Malaysian schools participated.