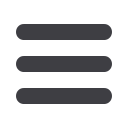
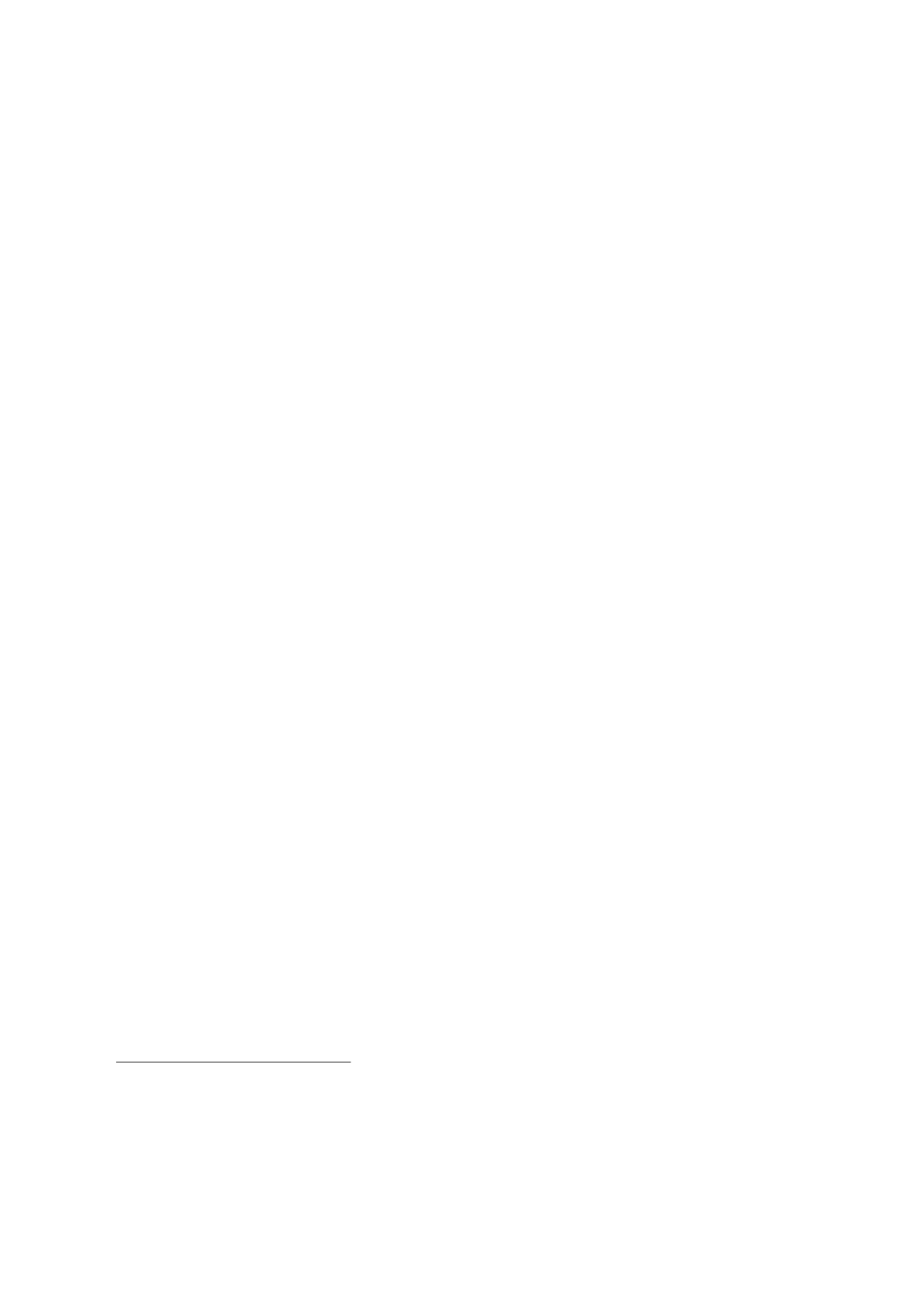
17
Third, not all student assessment exercises are conducted in the same year. Countries also vary
in terms of participation in a particular assessment round. The availability of data on input
quality indicators is also often specific to certain years. For these reasons, the composition of
countries for a given indicator for each given year can vary dramatically. Wherever possible,
five-year averages have bene used to ensure that comparisons of indicator averages are made
using the largest possible sample of countries.
In addition to looking at levels of learning outcomes, the analysis also comment on education
quality with reference to distributional concerns such as the extent of inequality in school
completion, inequality of opportunity in learning outcomes and the share of disadvantaged
students (i.e. those in the lowest 25% of socioeconomic status) who score among the top 25%
of students internationally, among students of similar socio-economic status. Detailed country-
level description analysis is performed with a focus on wealth groups. The wealth-learning
gradients are also compared over time and across countries.
Alongside using WIDE database of UNESCO and the World Bank’s World Data Indicators (WDI)
database of the World Bank, student level data has been used to study the determinants of
learning outcomes. Student level analysis of learning outcomes is primarily based on the fifth
(i.e. 2012) round of PISA survey where each student assessed had finished at least six years of
school. The methodological approach involves estimation of child-level educational production
motivated by factors recognized in the conceptual framework (Figure 1.1) which explain how
learners, educators and the schooling environment combine to produce learning outcomes.
Child specific factors also include pre-determined circumstances (e.g. early childhood schooling)
which predate current schooling choices. System-wide factors are recognized along with those
that relate to resources and accountability; subject to the underlying data set used, the model
specification accounts for governance issues.
For i-th student achievement score in a given subject (j) and country (k), the relationship
between inputs and output can be summarized in an achievement function as follows:
Student Achievement
ijk
=
f
(
C, F, S, I
) + e
ijk
where C, F, S and I are vectors of child, family/parent, school and institution specific
characteristics while e is the random error term. The regression function is estimated using the
ordinary least squares (OLS) technique. Vector C includes demographic factors such as the
student’s gender, whether the child has attended preschool or not. Vector F includes family
background variables used are also recognized as important circumstances factors and
characteristics over which adolescents have no control
such as
presence of parents at home,
education level of the most educated parent/guardian, immigration status, quintile in the
distribution of wealth, and city size.
10
The regression model is estimated using the ordinary least square (OLS) regression method.
PISA is used in lieu of TIMSS for two reasons. First, it assess student performance in three
domains whereas TIMSS is only limited to mathematics and science. Second, TIMSS data set
10
Balcazar, Narayan, and Tiwari (2015) employ the following factors to define the circumstances vector in their
research on inequality of educational opportunities using PISA data: (i) gender, (ii) whether the child has
attended preschool or not, (iii) presence of parents at home, (iv) education level of the most educated
parent/guardian, (v) immigration status, (vi) quintile in the distribution of economic, social and cultural status,
and (vii) city size.