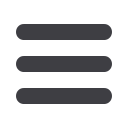
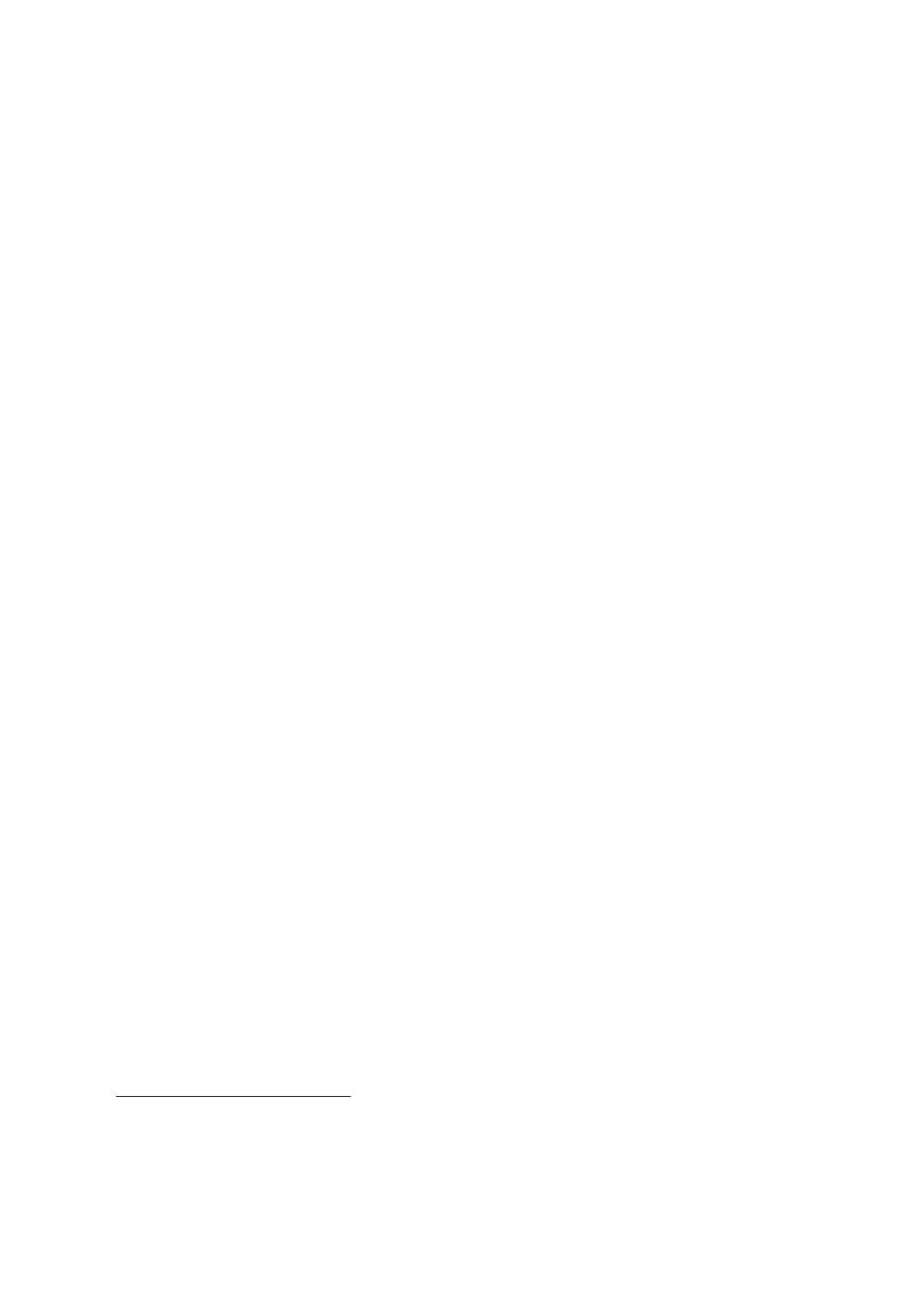
139
learning outcomes (though significantly less than in enrolment), with boys almost always
performing better than girls. These gaps are also persistent over the 2014-2016 period with
almost no noticeable improvement. Some regions continue to depict alarmingly wide gaps in
favor of males – FATA in particular (followed by Balochistan and KP) stand out as regions with
extremely high pro-male gaps in learning outcomes that are persistently wide in favor of males.
3.3.4.
Regression Analysis of the Determinants of Learning Outcomes
The descriptive statistics presented above hint at some key drivers of education disadvantage
in Pakistan and specifically in rural Pakistan (with gender, location and socio-economic status
among the most critical ones). A natural question to follow from this is whether these factors
are simply correlations or more deeply associated with learning outcomes? In order to arrive at
more nuanced and meaningful conclusions, this section undertakes simple multivariate
empirical analysis aimed at estimating some key determinants of enrolment and learning
outcomes for children in rural Pakistan.
This sub-section attempts to unravel the extent to which key factors, particularly that related to
household poverty, impact student learning. To do so, we take advantage of the rich ASER data
from Pakistan which assesses children in both literacy and numeracy and in doing so aim to
unravel some of the equity implications for example of going to a specific type of school, being
of a given gender or belonging to a specific region or socioeconomic class in the country. In order
to achieve this, ASER data from two years 2013 and 201639 are separately used to estimate
probit models to determine the link between a set of variables such as age, gender,
socioeconomic status (measured using the wealth index discussed previously) type of school
child attends, current class attended, parental education levels and region of residence
(province) on the likelihood of a child completing ‘higher-level’ learning for those enrolled in
school. Tables 3.3.1-3.3.2 report the results of the estimation for the full sample of children aged
5-16 years for the numeracy outcomes in 2013 and 2016 with columns further disaggregating
the results for the poorest and richest children (i.e. those in the poorest and richest quartiles).
The estimates for reading skills are presented in Tables 3.3.3-3.3.4.
There are some striking findings. Turn first to Tables on the determinants of numeracy
outcomes, for all children and those belonging to the poorest and richest quartiles in 2013 and
2016. Of the factors determining ‘higher-order’ numeracy skills, age and gender are clearly
important. Older children and those studying in higher grades are more likely to have higher
order maths skills. Male children are also more likely as compared to female children to achieve
more in numeracy and this appears to be the case amongst poorest quartiles (in 2013) and
amongst both poor and rich quartiles (in 2016) in the country suggesting a significant male
learning advantage. The wealth index is significantly positive for the full sample suggesting
socio-economic status positively influences learning outcomes in maths. Both parent’s
education also seems to positively influence maths outcomes and this doesn’t appear to differ
substantially across the wealth quartiles or over the years. Another striking finding is the
apparently better learning outcomes of children studying in ‘private’ schools as compared to
their counterparts in government, independent madrasah or ‘other’ schools. The magnitude of
the marginal effect is larger among the poorer children indicating that not only are poorer
children more disadvantaged by socioeconomic status but also double disadvantaged in terms
of achieving less when attending non-private schools.
39
The data from these years are used as it was from 2013 that ‘full’ district coverage for the entire Pakistan
because available with ASER data and these datasets provide the most comprehensive country-wide
information on learning in the country.